A recent study forecasts that AI language models will soon exhaust public data for training, presenting challenges for AI development. Researchers explore alternative data sources and specialized training techniques to address the data bottleneck, sparking both excitement and apprehension about the future of AI.
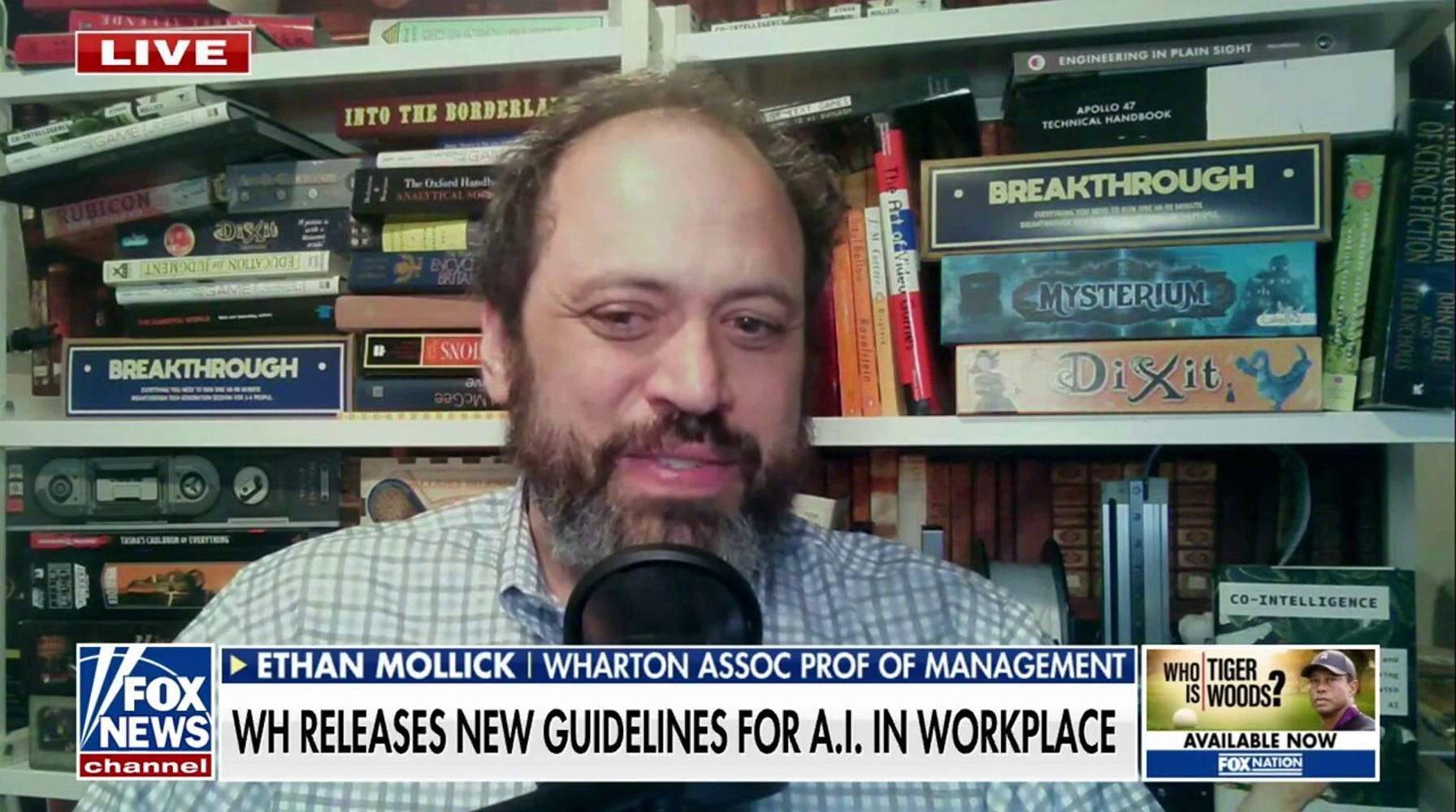
Artificial intelligence systems, such as ChatGPT, rely heavily on text data to improve their language processing capabilities. However, a new study by research group Epoch AI projects that tech companies will exhaust the supply of publicly available training data for AI language models by sometime between 2026 and 2032.
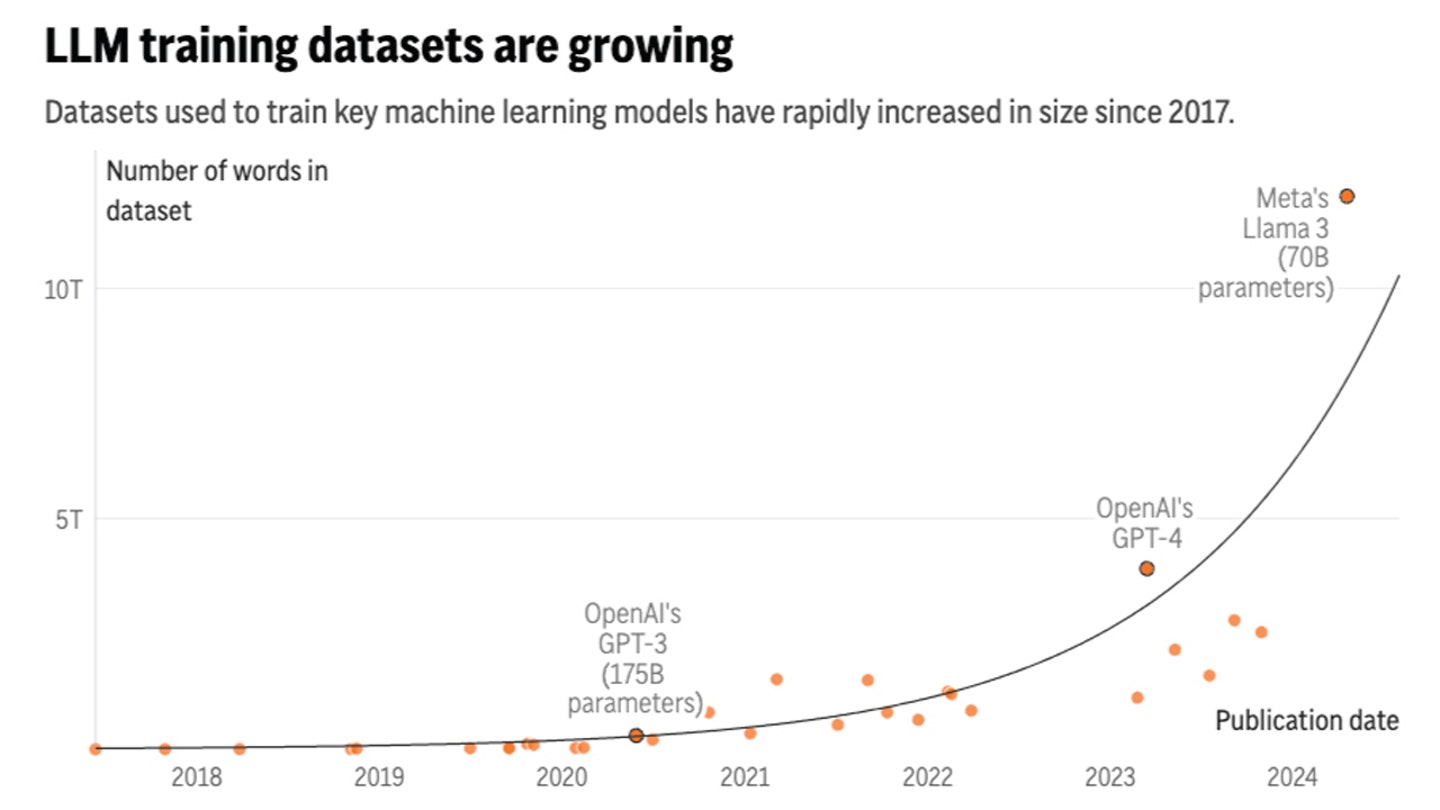
This data scarcity poses challenges for the continued development of AI language models. As Tamay Besiroglu, an author of the study, explains, "If you start hitting those constraints about how much data you have, then you can't really scale up your models efficiently anymore."
In response to the impending data shortage, tech companies are actively seeking high-quality data sources to train their AI models. Some have made deals to access data from Reddit forums and news outlets, while others explore alternative options.
In the long run, there may not be enough new public data to sustain AI development. Companies may resort to using private data, such as emails and text messages, or rely on synthetic data created by AI models themselves. However, both options raise concerns about privacy and data quality.
Besides training larger models, another approach to address the data bottleneck is to build more specialized training models for specific tasks. This requires developing training algorithms that can better utilize existing data and focus on specific domains.
While synthetic data can supplement real human-generated data, Nicolas Papernot, an assistant professor at the University of Toronto, cautions against over-reliance on it. "Training on AI-generated data is like what happens when you photocopy a piece of paper and then you photocopy the photocopy. You lose some of the information," he explains.
The impending data shortage has prompted discussions about the stewardship of data resources. Websites like Reddit and Wikipedia, as well as publishers, must consider how their data is used for AI training and ensure fair compensation and incentives for contributors.
Epoch's study suggests that paying humans to generate text data for AI training is not economically viable for driving better technical performance. AI developers explore alternative approaches, such as generating synthetic data, while recognizing the potential limitations.
The data shortage for AI language models has ignited both excitement and concern. Researchers seek innovative solutions, including specialized training techniques and alternative data sources. However, the ethical implications and limitations of synthetic data remain important considerations as AI technology continues to evolve.